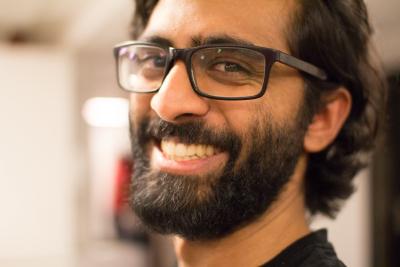
Alocias Mariadason
Alocias has a master’s degree in physics from the University of Oslo, submitted in spring 2018. The subject of the thesis was Quantum Monte Carlo Simulations of Quantum dots constrained in single- and double well potentials. He solved the numerical problem for both systems with new analytic expressions which had not been explicitly done before and a newer method with roots in machine learning to further improve upon the results.